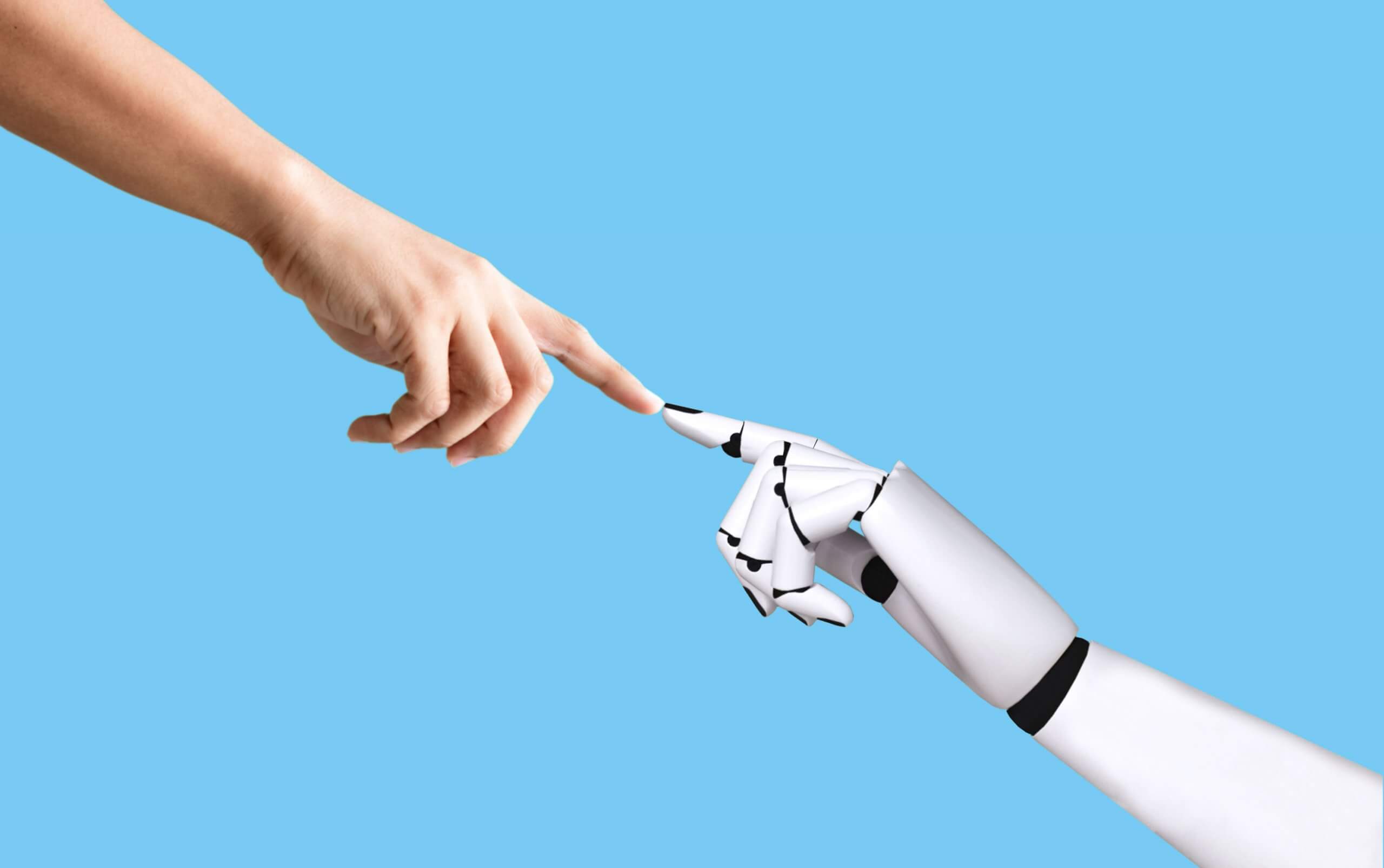
The Power of Automation: Unleashing the Full Potential of Logistics through Artificial Intelligence
From automation to real-time insights, explore how AI is reshaping logistics, empowering companies to gain a competitive edge in a rapidly evolving industry.
Introduction
Are you old enough to remember the Six Million Dollar Man? Today, we can harness the power of AI and automation to create a workforce of superhumans in logistics capable of delivering faster, more accurate, and more cost-effective solutions to customers around the world. By automating routine tasks, we can free workers to use their expertise to tackle new challenges and exceed expectations. With advanced machine learning algorithms and real-time data analysis, we can create a highly efficient and productive workforce, boost productivity, and ensure a more cost-effective and efficient workflow. It’s time to embrace the power of automation and bring logistics to new heights of efficiency and excellence. So let’s channel our inner Steve Austin and make logistics faster, better, and stronger than ever before.
This white paper aims to provide an overview of the critical considerations for players in the logistics and supply chain industries weighing to implement AI-driven automation. In addition, we will illustrate that path towards automation with multi-modal routing examples. The target audience for this white paper is logistics business owners, managers, and employees interested in learning more about how employing intelligent agents can benefit their organizations.
The scope of this white paper covers the specific types of AI-driven automation like machine learning (ML) and reinforcement learning (RL), their benefits and challenges, and best practices for introducing them in the logistics industry. Finally, we discuss what the future of AI in logistics may be.
As the logistics industry becomes increasingly complex and competitive, logistics companies need to understand the benefits and challenges of AI-driven automation and how to introduce it thoughtfully and strategically. This white paper aims to help logistics companies navigate this transition and stay competitive in an increasingly changing world by providing insights into the best practices for introducing automation.
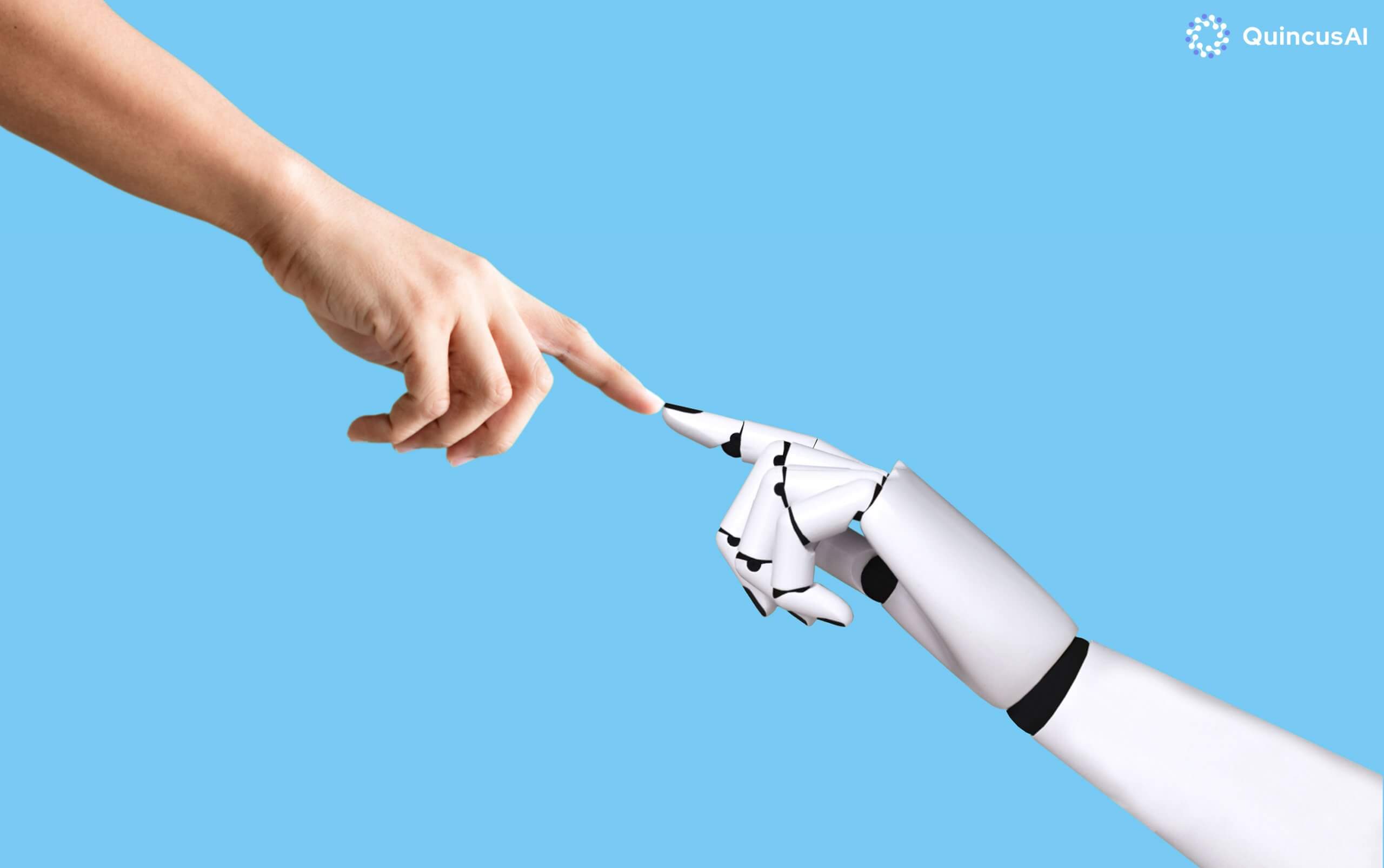
The benefits of automation
In today’s fast-paced business environment, companies constantly seek ways to increase efficiency and productivity while reducing costs and tackling sustainability issues (see our series on sustainability and AI). One of the most effective ways to achieve this is through automation. By automating routine and repetitive tasks, companies can save valuable time and resources while minimizing the possibility of human error. At Quincus AI, we take automation to the next level by using artificial intelligence (AI) as an automation vector.
AI to efficiently augment workers’ skills.
Our approach to AI is simple yet powerful: we “supercharge” workers by designing algorithms and building models that efficiently augment their skills. We believe any company’s value lies in its employees’ cumulative expertise and abilities. However, the increasing complexity of tasks due to the immense volume of data in all businesses makes it difficult for workers to focus on high-level work that requires critical thinking and creativity. Since the 70s, the business and social science literature (see References 1 to 5) has discussed the relationship between workload and mental strain and strategies for reducing it while promoting well-being in the workplace. We think the right AI tools will help workers thrive (notion described by Spreitzer et al. (2005)5) by broadening workers’ decision latitude that Karasek (1979)4 links to reducing mental strain.
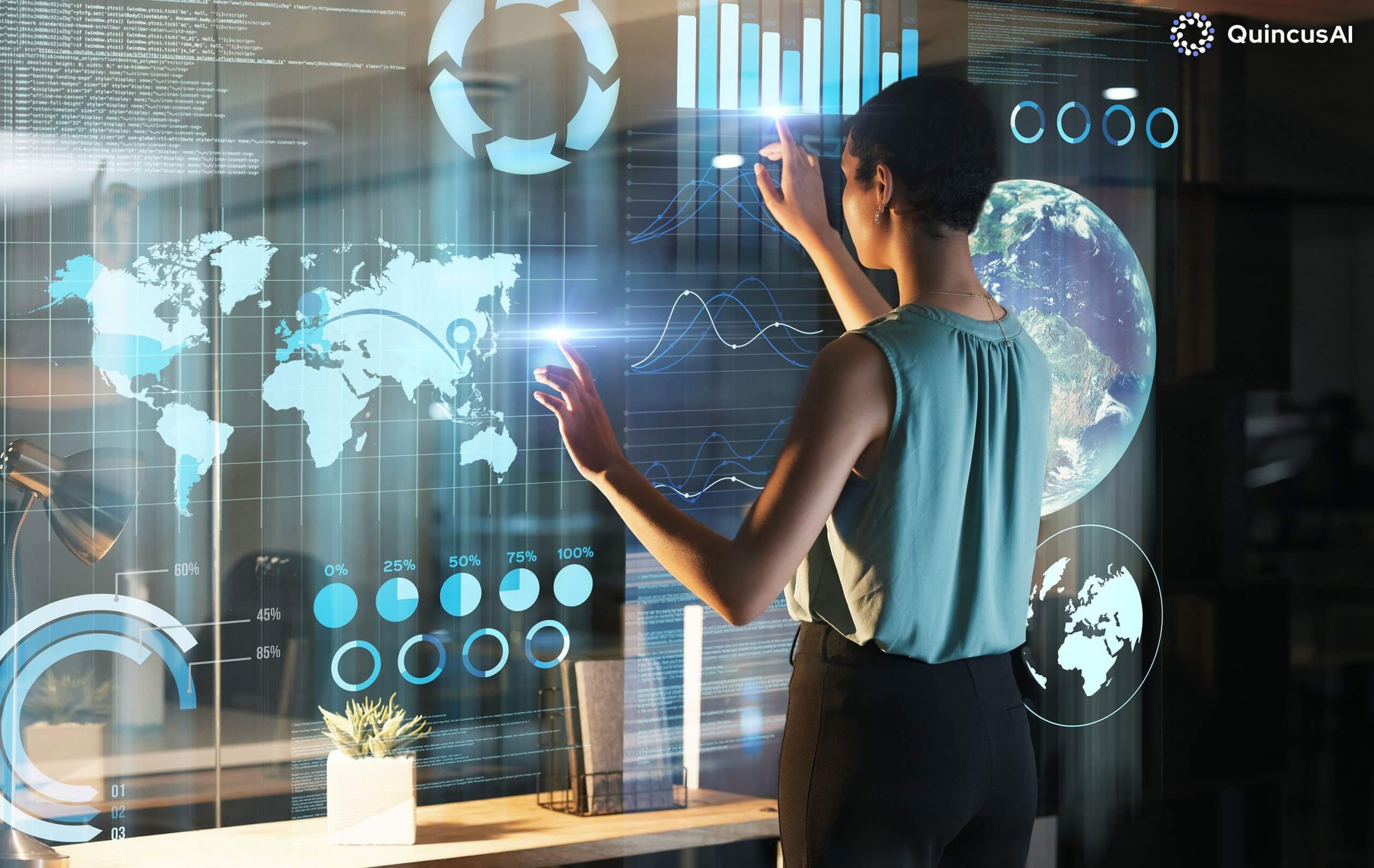
AI enables end-to-end visibility.
Automation can provide real-time visibility into supply chain operations, such as inventory levels, shipment tracking, and delivery status. This can enable logistics managers to proactively identify and address potential issues, such as delays or disruptions, leading to better supply chain performance. At Quincus AI, our vision is to build the metaverse of logistics where users would simulate operations for given periods in specific configurations. Then, different scenarios may be compared to decide what business model best suits particular situations.
We envision the metaverse of logistics as a digital twin of a logistical network. It is composed of four main elements, as shown in the below figure:
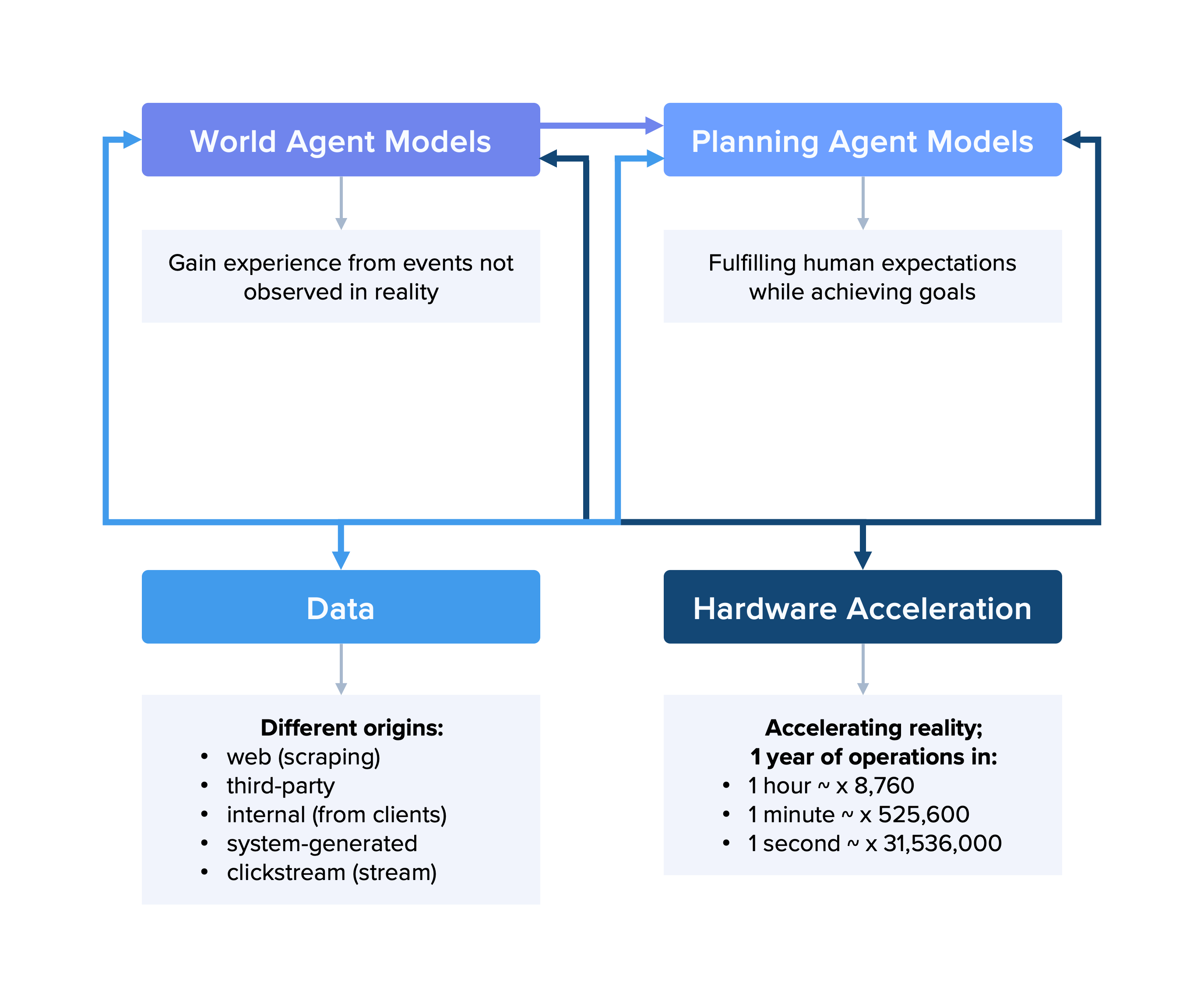
- Planning agent models: Our RL models are predictive and allow us to schedule and optimize transportation. The agents must behave as users expect.
- World agent models: We need to simulate the environment around our agents. We also use these models to learn from operations.
- Data: We feed these models with data. We need a high volume of data to be relevant and train our models.
- Hardware acceleration: Fast-forwarding operations require much processing power (GPU/TPU).
AI for data analysis
Automation can collect, process, and analyze large volumes of data. This can provide insights into key business metrics, such as delivery time, carbon emissions, or costs, which can inform strategic decisions and drive business growth.
Case in point: the logistics industry
In the logistics industry, dispatchers deal with thousands, if not millions, of packages per day to send worldwide through multiple hubs and with various transportation modes under tens of constraints like short time windows or priority levels. While dispatchers use heuristics they’ve built through years of experience, unplanned events can render their best practices and templates irrelevant, causing them to face situations they don’t know how to tackle. That’s where our RL-driven automation comes in.
When dealing with logistics and supply chains, unforeseen events can disrupt operations and confuse dispatchers. At Quincus AI, we use state-of-the-art RL models to help them. We chose that technical option by analogy with the autonomous vehicle field, where a self-driving car must learn to react to any event, even those never encountered before.
RL is a type of machine learning where an agent learns to make decisions by interacting with an environment and receiving rewards or penalties for its actions. The agent may observe what happens in that ecosystem only partially and may need to cooperate with other agents to achieve the best overall outcome.
With RL agents to support their decisions, dispatchers get real-time feedback and information, generate metrics to objectivize their new strategy, and make informed decisions—all that, of course, in a far shorter time. They can even boost their expertise because they increase the scope of alternative tactics they see and use.
We go beyond by using multi-agent reinforcement learning (MARL), where multiple intelligent agents learn to cooperate. For the company’s overall benefit, agents may “sacrifice themselves” and be individually sub-optimal as long as, globally, the dispatch of all packages is optimal. MARL is vital in logistics and supply chain management, where dispatchers must consider multiple correlated factors to find optimal solutions.
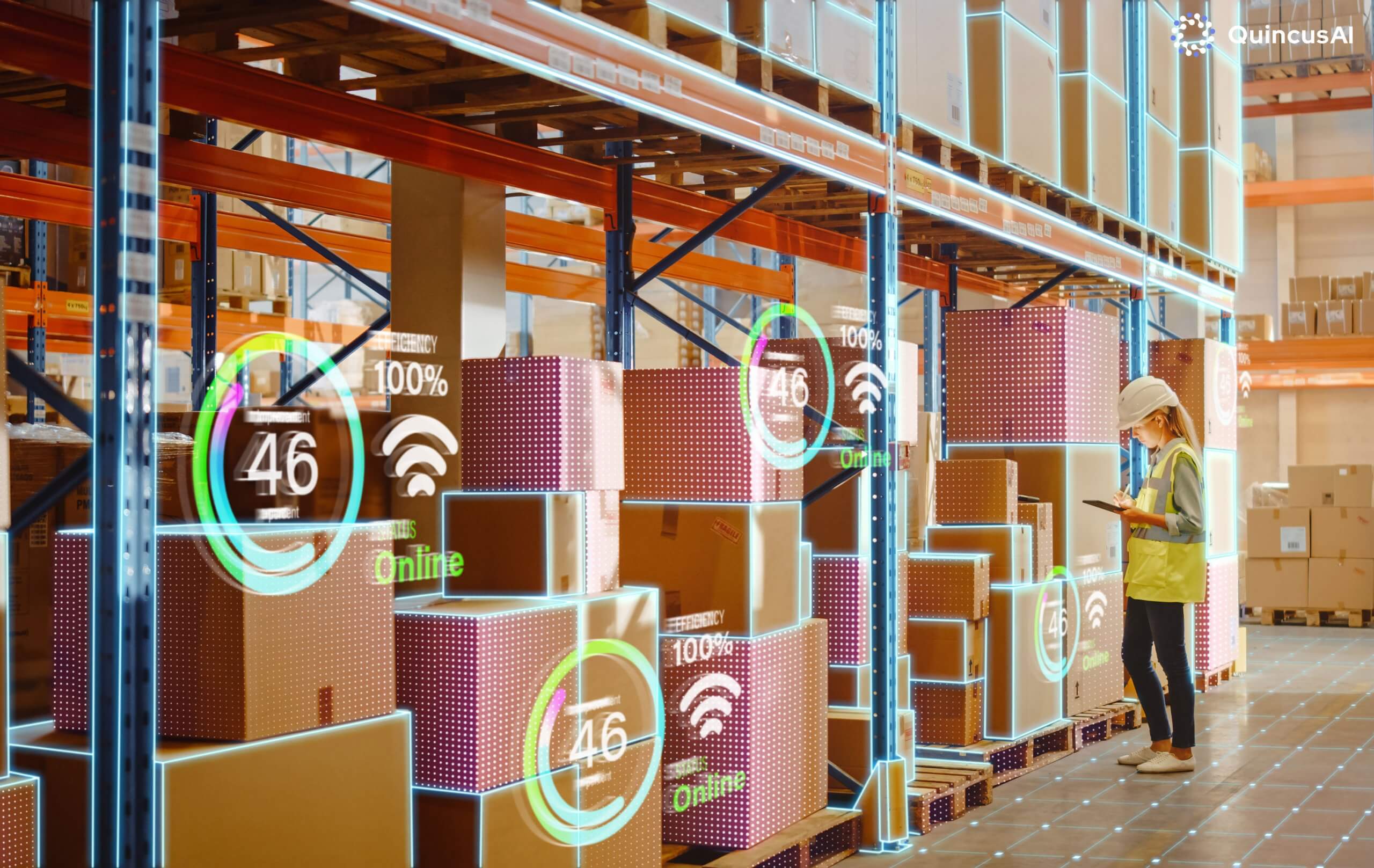
Automation Challenges: Breaking Through Barriers
Although building AI models can revolutionize logistics, there are hurdles to overcome. Bias poses one of the most significant threats, leading to inaccurate predictions and subpar solutions. This is especially true when algorithms are trained on incomplete, incorrect, or biased data. Logistics data is abundant, but the risk of biases increases without proper collection and cleaning processes.
Moreover, creating and training AI models requires massive data and computing resources (GPU, TPU), resulting in high data acquisition and treatment costs. These costs present a significant barrier to entry for small and mid-size businesses, increasing initial implementation costs.
Users present another obstacle. The gap in skills is vast, ranging from data scientists and ML engineers to those with no experience managing AI systems. A plug-and-play AI tool is essential to ease the first phase of automation. It must be robust and flexible enough to augment the expertise of novices and savvy users, empowering users to take charge and utilize its power to enhance their skills.
Best practices for introducing automation
Human beings overcome technical limitations and biases
At Quincus AI, we overcome technical hurdles and biases by conducting user-centric applied research (see our approach to design thinking). By tapping into the design fiction6 framework, we dig out the most reasonable and equitable assumptions to address our users’ challenges. We don’t just work for users; we work with them and owe our successes to them.
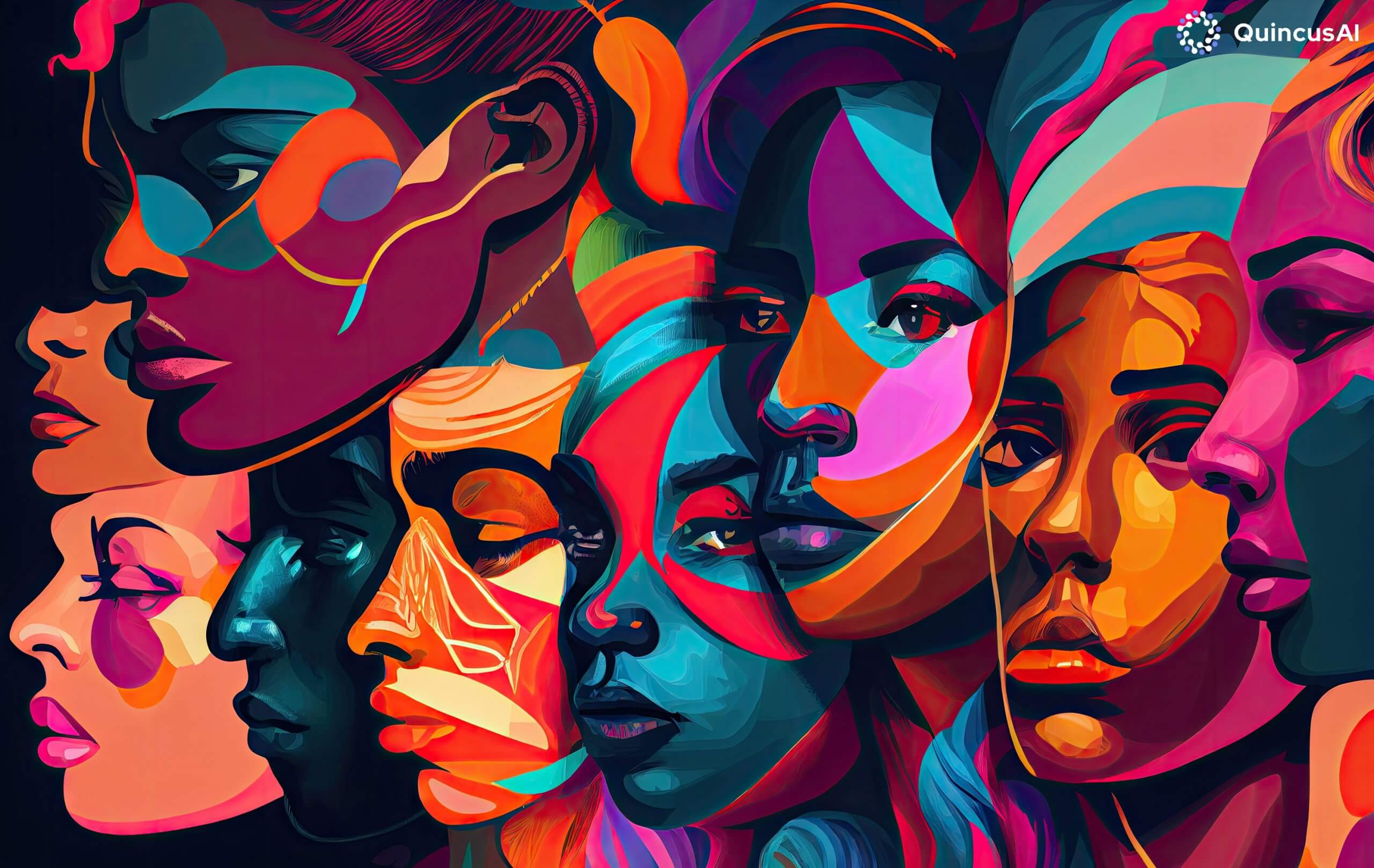
When we develop ML and RL models, we realize that AI is no child’s play; it’s a serious science that demands attention to detail. Data scarcity, acquisition hurdles, and unreliability pose challenges we must overcome. Mathematical and computing limitations also come into play, making it harder to achieve convergence. To make the most out of this situation, we need to take a few shortcuts, but we must be careful not to make hasty decisions.
That’s why we place the user at the core of our research, allowing us to uncover the most relevant and unbiased assumptions that can drive our products forward. By putting ourselves in our users’ shoes and identifying their pain points, we can make quick, informed decisions that propel us in the right direction.
We can also decide faster which technical options to adopt. For instance, transportation systems are built mainly around the hub and spoke design. As a result, the topology of the transportation network follows specific patterns that influence how we approach machine learning. To build a robust transportation network, we use a combination of imitation learning and reinforcement learning. Imitation learning allows us to train agents from a shared policy, which enables them to move seamlessly through dense areas following the same policy. This approach works well because most routes have mainstream parts and go through big hubs with many in-and-out connections. However, when we encounter zones that require more explorations and alternatives, we switch to reinforcement learning. By using a more complex and subtle RL policy, we can discover new ways to optimize parcel routing.
This approach helps us balance efficiency and innovation to create the best product for our users.
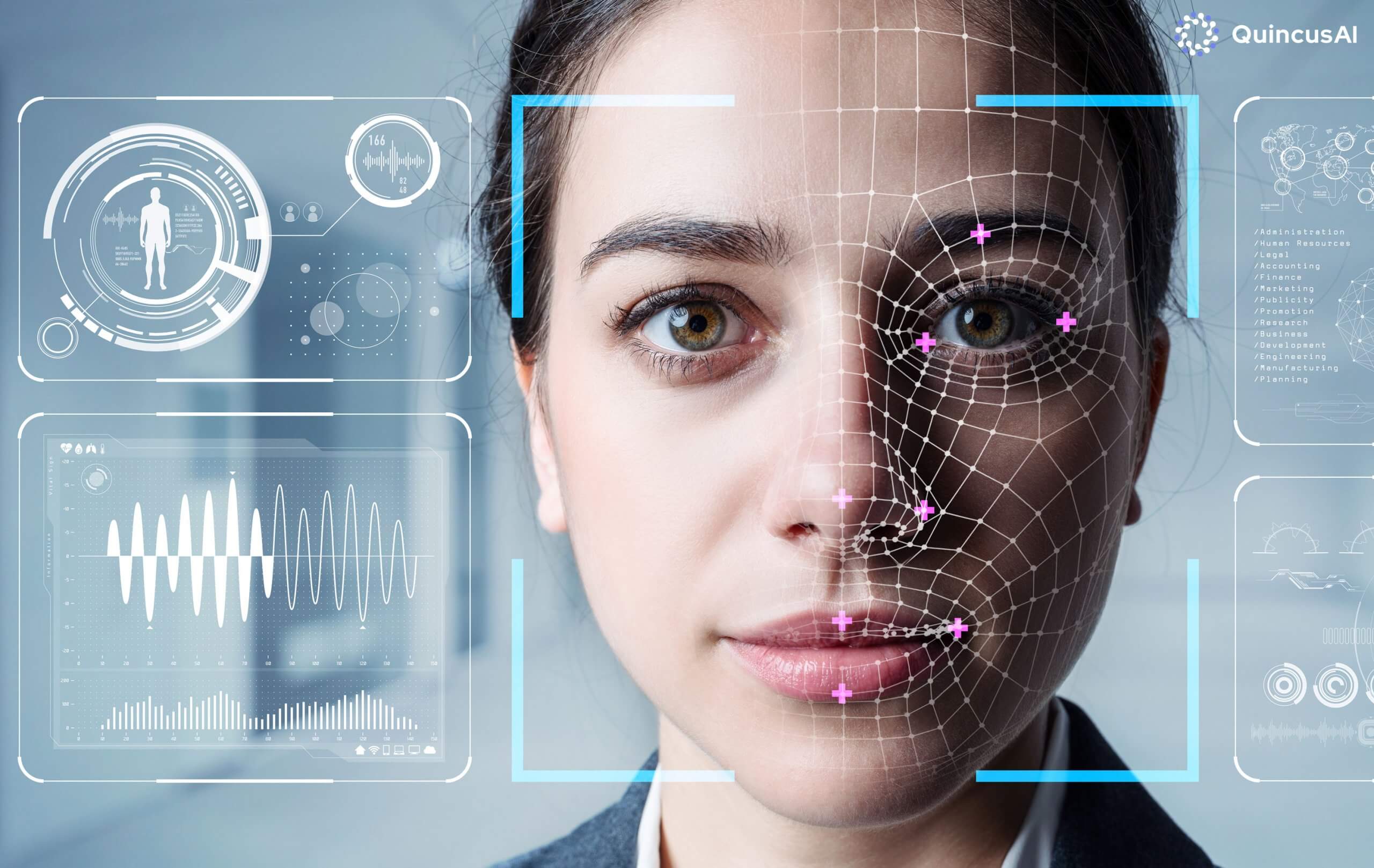
Starting small but steadily
In the world of business, both persistence and dynamism are desirable traits. Still, when it comes to R&D spending, persistence reigns supreme. The reason for this is something called the gestation period. When R&D spending follows a seesaw pattern, it leads to inefficiency, unproductive phases, and a lag in productivity. In contrast, persistent spending allows for a steady flow of new knowledge and optimal productivity. Pennetier et al. (2019) found that in realistic scenarios, persistent spending can improve R&D output quantity by 5 to 10 percent and quality by up to 30 percent, compared to dynamic spending. In short, persistence is critical to unlocking long-term success.
To smoothly transition into AI-driven automation, companies should take a persistent approach. As the saying goes, “slow and steady wins the race.” To make it happen, consider the following steps:
- Pinpoint where automation can shine, such as complex, technical, error-prone, time-consuming, and repetitive tasks.
- Define what machines and humans will do, such as computing optimal solutions, generating more alternatives, and decision-making. This clarification will help everyone understand the AI and automation project’s purpose and advantages.
- Start small: Begin by automating existing tasks to acclimate employees to the technology and boost their confidence in its effectiveness. This phase will also help identify and resolve problems before moving on to more complicated and creative tasks.
- Get employees on board: Involve them in co-designing the automation process, communicate its benefits, and provide training and support to adapt to the new methods.
- Monitor and measure results: Track progress and ensure the automation project achieves the desired outcomes.
- Evaluate and adjust: Review the automation project results regularly. Make adjustments to align with the company’s goals and maintain desired results.
With these steps, companies can gradually introduce automation, reduce disruption, and ensure a smooth transition, remembering that “Rome wasn’t built in a day.”
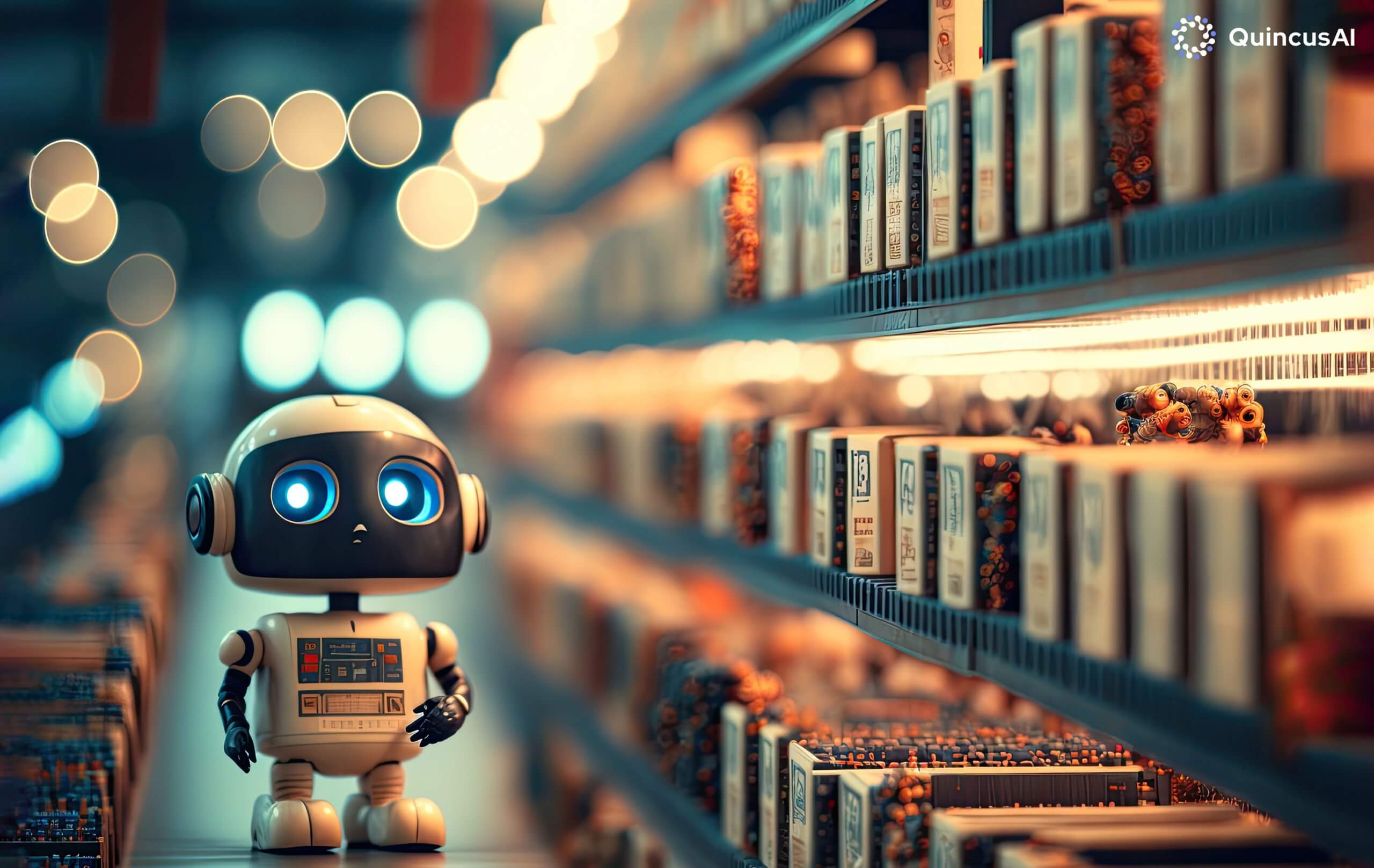
Conclusion
In conclusion, this white paper highlighted the benefits of AI-driven automation in logistics and supply chain management. AI in automating routine tasks can free workers to focus on high-level tasks that require critical thinking and creativity. Additionally, AI can provide the following:
- Real-time visibility into supply chain operations.
- Enabling logistics managers to identify and address potential issues proactively.
- Leading to better supply chain performance.
AI for data analysis can also provide insights into key business metrics and inform strategic decisions that drive business growth. The Quincus AI approach to AI is to design algorithms and build models that efficiently augment workers’ skills. This white paper has provided an overview of the types of AI-driven automation, their benefits and challenges, and best practices for introducing them into the logistics industry. The future of AI in logistics looks promising, with the metaverse of logistics composed of planning agent models, world agent models, data, and hardware acceleration, creating a highly efficient and productive workforce that delivers faster, more accurate, and more cost-effective solutions to customers around the world. Logistics companies embracing automation and AI’s power stand to gain a competitive advantage in an increasingly changing world.
References
- Demerouti, E., Bakker, A. B., Halbesleben, J. R. B., & Schaufeli, W. B. (2009). Work-related burnout and job engagement: A meta-analytic approach. Journal of Vocational Behavior, 74(3), 97-117.
- Grant, A. M., & Parker, S. K. (2009). Redesigning work design theories: The rise of relational and proactive perspectives. Academy of Management Annals, 3(1), 317-375.
- Hakanen, J. J., Bakker, A. B., & Schaufeli, W. B. (2006). Burnout and work engagement among teachers. Journal of School Psychology, 43(6), 495-513.
- Karasek, R. A. (1979). Job demands, job decision latitude, and mental strain: Implications for job redesign. Administrative Science Quarterly, 24(2), 285-308.
- Spreitzer, G. M., Sutcliffe, K. M., Dutton, J. E., Sonenshein, S., & Grant, A. M. (2005). A socially embedded model of thriving at work. Organization Science, 16(5), 537-549.
- Aguesse, M., & Decreton, B. (2022).Using Fiction to Find Your Strategy, Harvard Business Review.